A brain-inspired generative foundation model of primate locomotion
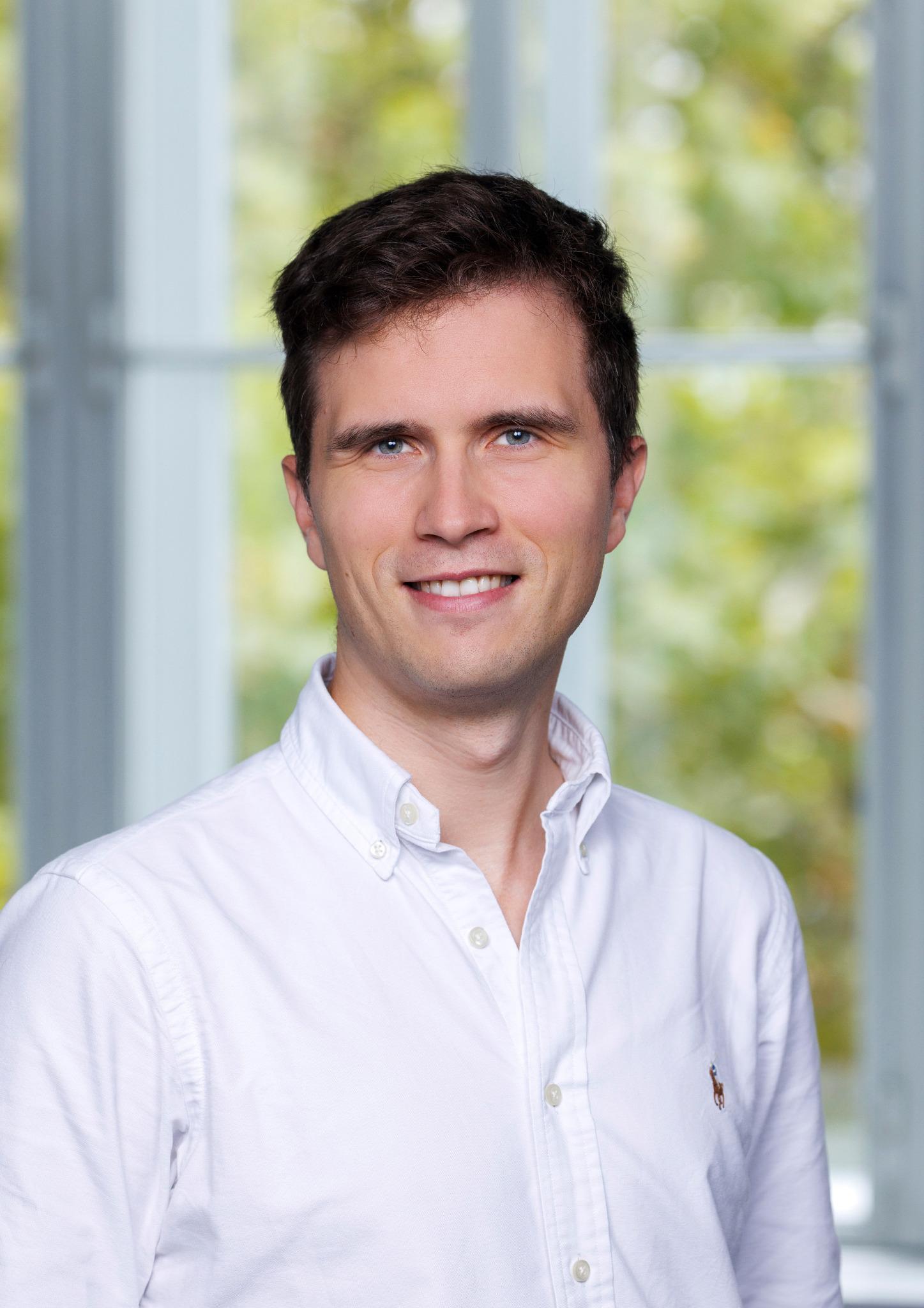
>>Context
Neuroprosthetic devices can restore functional movements for individuals with paralysis and other motor disorders. However, they require extensive training and demand a high mental load, even for basic functions such as walking or grasping. This is in part because current artificial neural controllers diverge from the brain’s hierarchy of motor control. Normally, the motor cortex predominantly focuses on complex goal-oriented behaviours, while lower-level structures, such as the cerebellum and spinal cord, coordinate simple movements. In contrast, patients with neuroprosthetic devices essentially re-adapt the neural activity in their motor cortex to control basic motor actions such as moving a leg.
>>Objectives
We propose that generative machine learning (ML) models for locomotion can bridge high-level goal-oriented signals in the motor cortex with low-level muscle activations in the spinal cord while accounting for sensory feedback. Such models could bring the next breakthrough in neuroprosthetics, enabling effortless and adaptive locomotion.
We will develop a generative Foundation Model (FM) for locomotion that can generate diverse locomotor behaviours controlled by high-level neural signals recorded from the cortex, while flexibly adapting them to the physical environment based on sensory feedback. This research relies on the mathematical formulation of a new class of ML models, which we combine with large-scale datasets of simultaneous single-neuron recordings, high-speed video-based kinematics and muscle activations in non-human primates during cognitive decision-making and locomotion tasks. We have early access to this data through my established collaborations with Profs. Mehrdad Jazayeri at MIT and Gregoire Courtine at EPFL, and other experts in the field. The project is organised in three aims:
Aim 1: Formulating a predictive ML theory based on brain-inspired compositional generalisation.
The brain represents simple movement ‘primitives’ as dynamical processes involving neuron populations and rapidly combines them to perform new tasks with zero-shot or few-shot learning. Based on our extensive preparatory work on ML for neuronal dynamics, we will mathematically formulate a new class of ML models that can reconstruct the neural representations of such movement primitives from sparse neural recordings and, from them, predict the neural dynamics during complex locomotor movement through compositional generalisation.
Aim 2: Developing a generative FM of low-level primate locomotion.
While generative FMs have been successful in natural language processing, they cannot readily solve robotics tasks such as locomotion due to the high dimensionality and dense interaction with the environment. We will use offline reinforcement learning to formulate locomotion as an optimisation problem on multiple time horizons and establish a latent representation of corresponding goals over these time scales. We will show that locomotion can be modelled as an autoregressive prediction problem in this latent space, taking inspiration from natural language prediction.
Aim 3: Predicting low-level motor patterns controlled by high-level cortical recordings.
We will align the latent representation of neural signals inferred in Aim 1 and movement goals inferred in Aim 2 to obtain a generative FM that is controllable by high-level cortical signals. This model will allow us, for the first time, to predict complex motor actions using neural recordings of high-level processes in the motor cortex. Our investigation will disambiguate the synergistic role of the cortex and lower-level motor circuits in controlling locomotor tasks of varying complexity. Finally, we will use our model to generate muscle activation signals during locomotion to provide a proof-of-principle for using our method in brain-spine neuroprosthetics for locomotion.
>>Impact
This project is a major step toward ML-based methods with brain-like generalisation of skills, broadly relevant for neuroprosthetics and the wider field of robotics. To realise this impact, we will establish long-term collaborations with leading researchers in neurorehabilitation, including Prof. Oskar Aszmann and Karen Minassian at the Medical University of Vienna. Finally, our ML modelling of locomotion puts key hypotheses about hierarchical control and the neural basis of movement to a test, and establishes an in silico testbed for hypothesis testing with reduced need for invasive animal experiments.