Robotic Action-Language Learning through Interaction (RALLI)
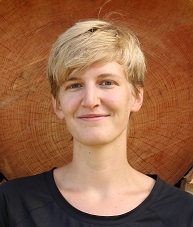
In the last few years, the demand for installing robots to support and collaborate with humans in day-to-day tasks or in industries has rapidly increased. Developers of robots, however, are not able to foresee all upcoming situations the robot might encounter. Therefore mechanisms are required that allow the robot to learn new tasks from observations and verbal descriptions from a human tutor. Our approach is inspired by insights from developmental language learning. In caregiver-child interactions, typically objects are shown and actions performed, while the tutor is uttering what they are doing. In addition, objects are brought onto the learner’s field of visual attention.
In order to investigate and analyze the characteristics of human task descriptions in more detail, we first collected data of people conducting a task and describing what they are doing. We then tested different models and learning approaches, and implemented and tested the most successful ones on a robot. It could be shown that mechanisms inspired by human language learning are very useful to enhance computational language learning and to successfully learn from small input data. This differs greatly from prevalent computational learning approaches that rely on large datasets.
Thus, the results of the project lay the foundation for developing future robots being equipped with the possibility to acquire new tasks and behaviors on the job both through observation and through natural language instructions. There exists a broad variety of possible application scenarios and they all have their own requirements. In home and health care applications, learning specific subtasks that are embedded in larger personal contexts is important. This includes, for example, the requirement for a care robot to recognize and adequately react to (non-verbal) human signals to identify their needs. A home assistant robot has to be able to differentiate between actionrelated communication (e.g. pointing at an object to put it in focus) and meta-communication (e.g. gesturing to emphasize the utterance in general). This is important in order to identify those sequences of multimodal input the robot can learn from.
Other than in the private context, the type of interaction in industries is more focused on efficiency and productivity, as industrial robots are primarily needed and designed for enhancing industrial processes. In addition, the robot’s body, its perception systems and possibilities to move, also determines how it is able to conduct actions and communicate with the human.
In all of these application scenarios, the insights gained from the research questions tackled in Ralli and the integrated robot system developed in Ralli can serve as a basis for implementations of those aspects of robotic systems where situated (inter-)action plays a role. During the course of the project, especially the demand of industries has increased for robots collaborating with workers and for teaching robots new tasks similar to apprentices. In two follow up projects (“Tutoring of robots in industries” (WWTF project NXT19-005) and “CoBot Studio” (FFG project 872590), we thus build on results from Ralli and focus on worker-cobot interaction in industries.